The Future of Data Science: Trends to Watch in 2025
From AI to quantum computing breakthroughs, the future of data science is rising with great potential. So, if you’re wondering where your future lies, it’s here! With uncertain changes how can you stay ahead in this dynamic field of your interest? Let’s clear all your doubts and give you a proper understanding of the future of data science and the latest trends. Irrespective of your experience, you should stay updated and informed about the trends to remain relevant and current in your field. Let’s get started.
What is data science and why is it in demand?
In simple words, Data science is the study of data to manage perceptions and build strategies. Data scientists analyse the data and use it to develop a data-driven solution. This is applied across various industries to build effective strategies and stimulate development, which is the main reason for its demand. Data science has become a vital part of most industries, driving innovations and decision making. Let’s explore some of the reasons for its widespread interest.
Improved Business Value: This helps in business development, identifies potential revenue streams, optimises operations, and reduces costs.
Solution Provide – Data Science helps solve complex challenges by analysing databases, predicting trends, and identifying different patterns.
Better Customer Experience – Aid for better customer targeting and also better product development.
Stronger Scientific Research – Helps in advanced search, by predicting options and models.
Now, let’s explore some of the progression in the future of data science.
Edge Computing in Data Science
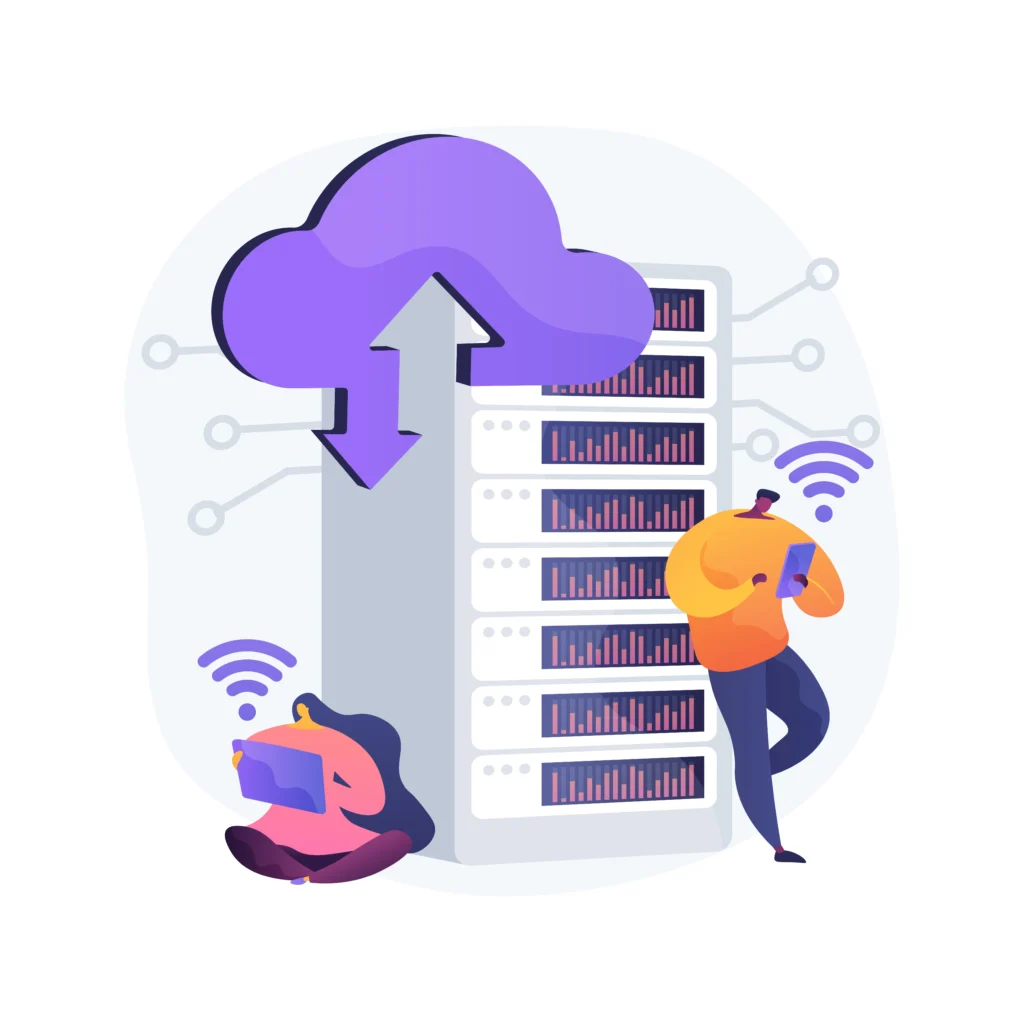
The edge computing is reshaping the landscape of data analysing and processing significantly influencing the future of data science. The development is making close observations on how we observe and interpret data.
Reduced Data Processing Latency – As edge computing brings data processing closer to the source, it helps reduce latency. It enables more efficient data collection and pre processing which reduces usage of bandwidth, data storage costs, and better data quality and relevance.
Realtime Analytics – Edge computing allows for real-time analytics to be performed directly on IoT devices or nearby edge servers. This capability opens up new possibilities for immediate insights and actions. Edge computing helps in distributed processing, zero latency, real-time processing, and limited connectivity.
As we go ahead, the integration of these technologies with other emerging trends will further shape the landscape of data science.
AI Integration in Data Science
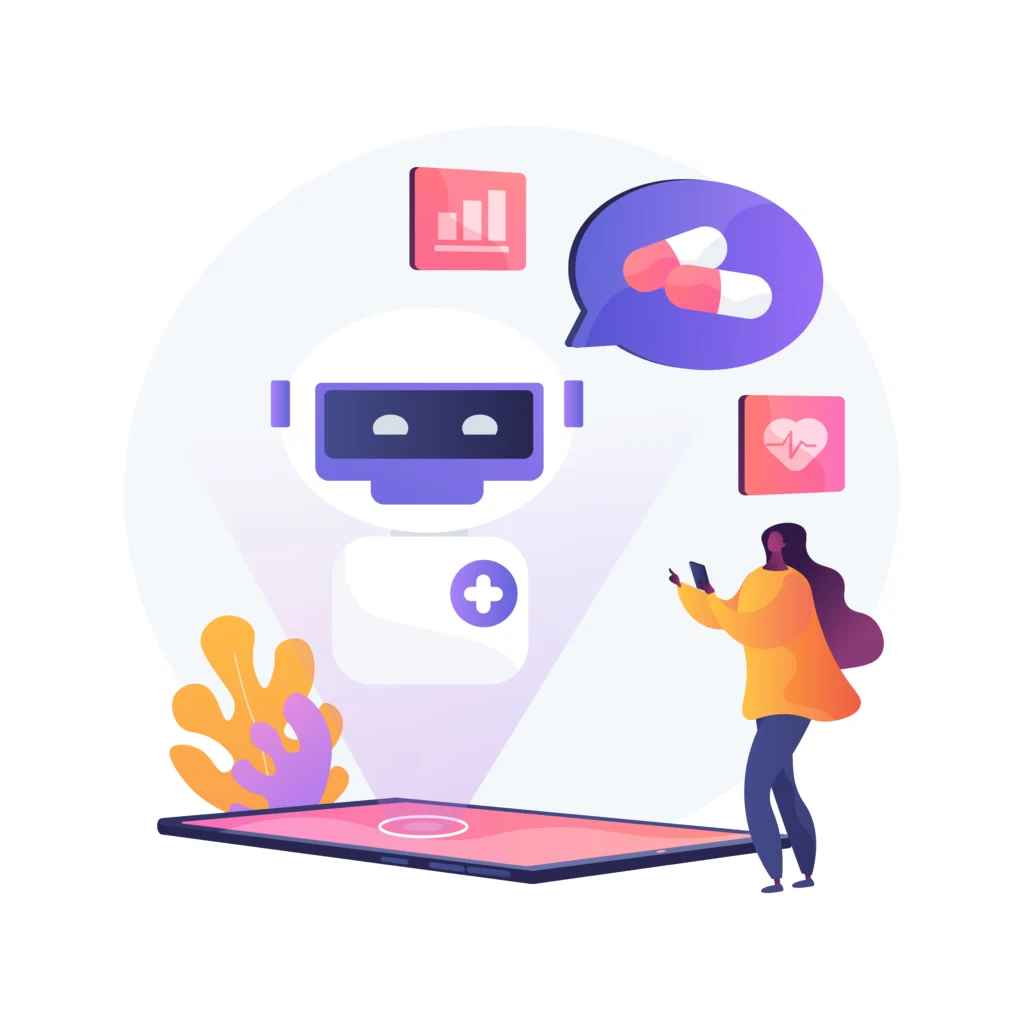
As data science grows alongside artificial intelligence, their combined application is becoming more prevalent. It is enhancing technical operations and opening doors to more possibilities.
Advancements in Machine Learning
Machine learning, a sub-category of AI, is experiencing rapid advancements that are changing data science practices. These developments are providing more understanding of complex databases, helping,
- Deep Learning – Neural networks are getting better, making it easier to recognise patterns in large datasets, and making improvements in natural language processing(NLP). Models like GPT-4 and BERT have improved how machines understand and generate human language.
- Boost Learning – This approach is becoming popular in situations where decision making needs to improve over time. Innovations in this field are leading to advancements in robotics, game playing, and autonomous systems.
- Learning Application – Using knowledge from one task to improve another is making models more efficient and reducing training time.
Natural Language Processing Developments
Natural Language Processing (NLP) is improving, making it easier to understand and generate human language.
Transformer Models – Large language models like BERT, GPT-3, and GPT-4 are developing text analysis and generation. It has also introduced zero shot and few shot learning, where models can perform tasks, with little to no task specific training data by using their general language understanding.
Sentiment Analysis – Advanced algorithms are providing more accurate emotional context from text data.
Multimedia Models – Models that function with other data along with NPL, are becoming more in use. These models, like OpenAI’s CLIP, can understand and generate content differently.
These AI integrations are not only boosting data scientist’s capabilities but also shaping the future of data science by making advanced analytics more accessible to a wider range of professionals.
Ethical AI and Responsible Data Science
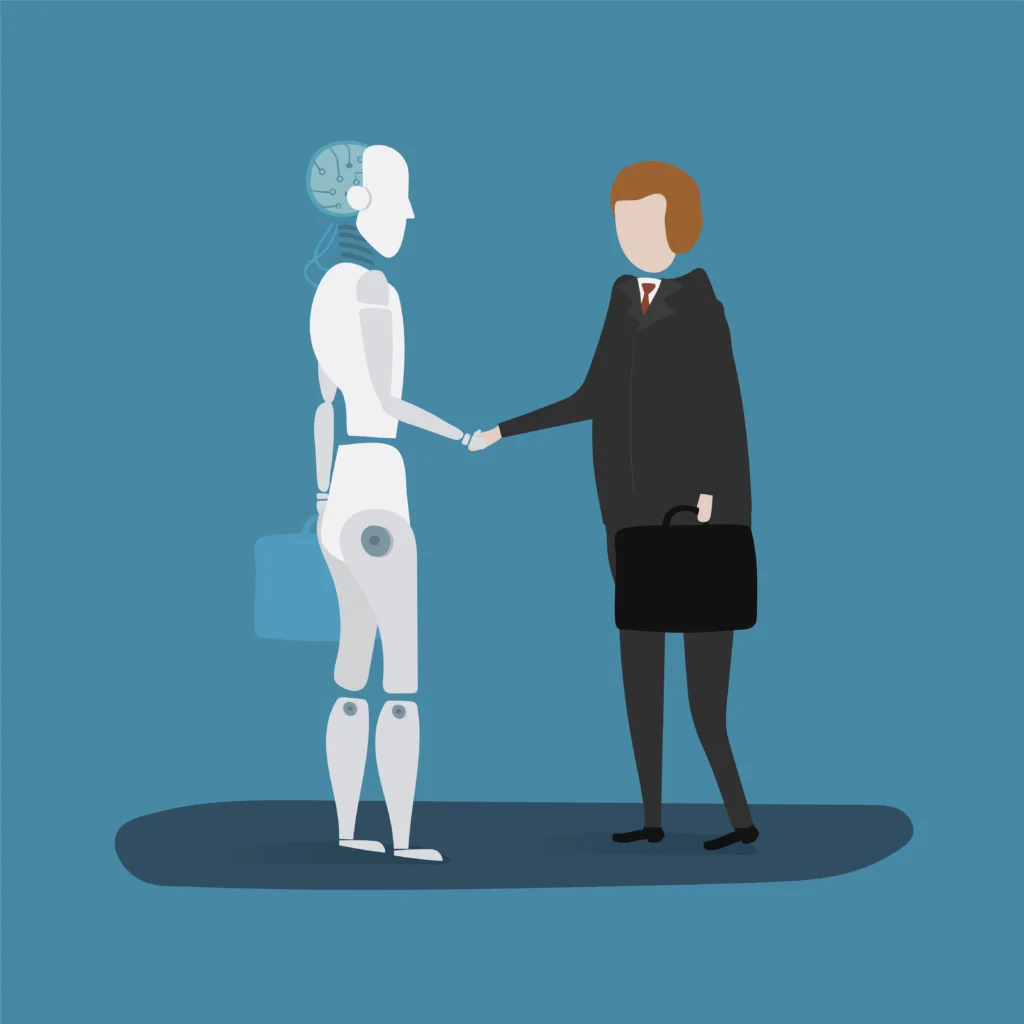
As technology evolves, maintaining its ethical use is essential to ensuring the quality of future data science. In 2024, there will be more focus on ensuring that AI systems contribute positively to the future of data science by being powerful, fair, transparent, and respectful of privacy.
Data Privacy Enhancements
With growing concerns about data breaches and misuse, now we will see some strong advancements in data privacy technologies, which will be the deciding factor for the future of data science. Some of these include,
- Collaborative Learning – Allows model training on decentralised data.
- Differential Privacy – Adds noise to data to protect individual privacy.
- Homomorphic Encryption – Can enable computations on encrypted data through techniques like Secure Multi-Party Computation (SMPC) and Homomorphic Encryption. These methods allow data to be processed and analysed without exposing the raw data, enhancing privacy and security.
Fairness in AI Algorithms
Fairness in AI is a priority. Key developments include,
- Pre-processing techniques to balance training data.
- In-processing methods improve fairness during model training by adjusting the process to reduce biases and ensure fair results.
- Post-processing approaches adjust model outputs to ensure fairness.
Data scientists will use fairness tools more to find and fix bias in their models, helping to prevent AI from making inequalities worse.
Automated Machine Learning (AutoML)
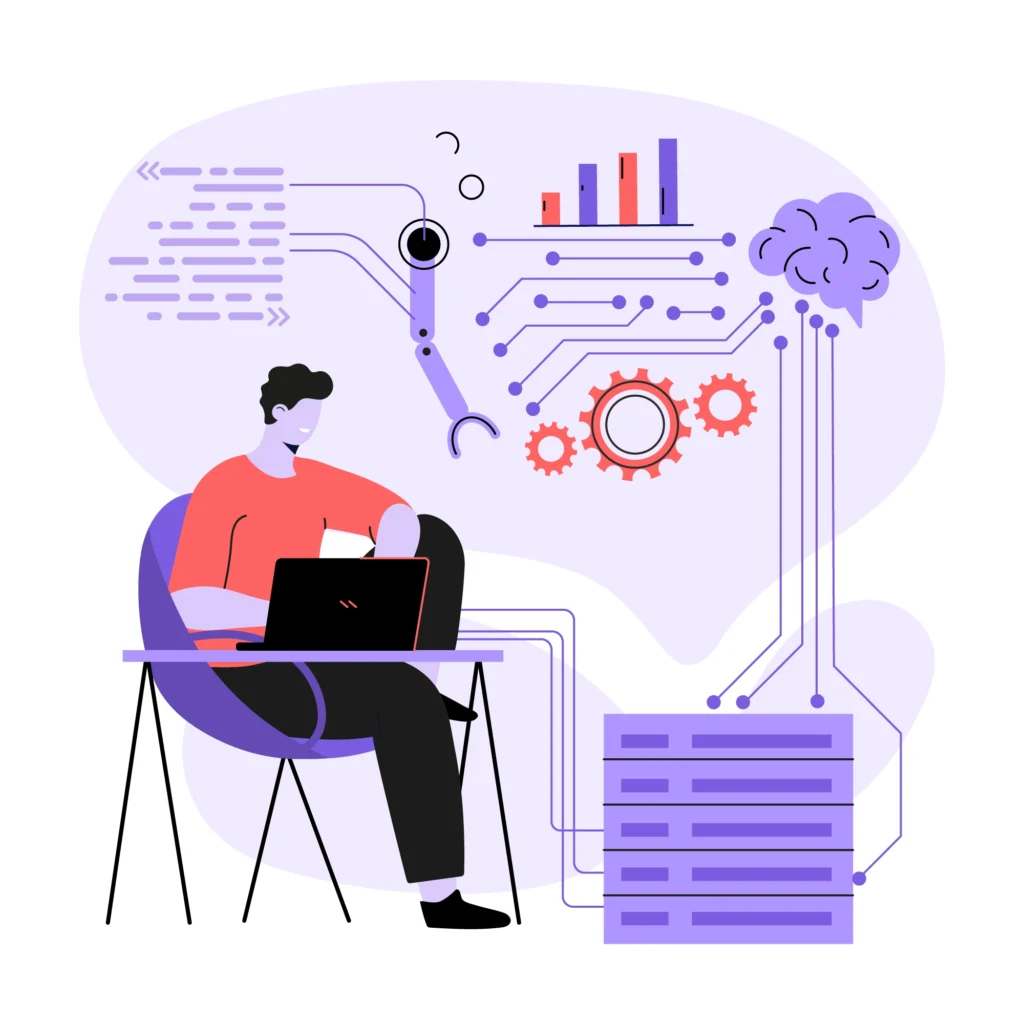
Automated Machine Learning, or AutoML, is improving data science by simplifying and accelerating the process of building and using machine learning models, playing a key role in shaping the future of data science.
Improved Model Accuracy – AutoML structures automatically choose, adjust, and enhance machine mastering models, frequently getting better results than guide techniques. They clarify models, combine multiple models for better performance, and handle feature selection and creation.
Reduced Need for Expert Data Scientists – While AutoML doesn’t replace data scientists, it makes machine learning easier for non-experts and lets data scientists focus on more complex tasks. Instead of manually selecting models and tuning parameters, AutoML self-processes these and discovers features quickly.
Faster Model Development—AutoML speeds up building and using machine learning models, helping businesses quickly adapt to changes. It also lets you test models faster and tune model settings more efficiently.
Building and using machine learning models of Data Science – AutoML makes machine learning more accessible by letting domain experts build models without deep ML knowledge, enabling smaller companies to use AI without large data science teams, and encouraging more people to experiment with ML. In the future, AutoML will continue to advance with new techniques, further improving its impact on data science.
Data Science in Cloud Environments

Cloud environments are changing data science by providing better flexibility, teamwork, and the ability to handle large-scale data. Here are the key aspects of working with data science in the cloud.
Integration of Cloud-Native Tools – Cloud-native tools are designed for the cloud, offering smooth integration and high performance. Key tools for data scientists include:
- Data Storage: Databases like Amazon DynamoDB and Google Cloud Bigtable.
- Data Processing: Serverless services like AWS Lambda and Azure Functions.
- Machine Learning: Managed services like Amazon SageMaker and Google Cloud AI Platform.
Collaborative Data Science Platforms – Cloud-based platforms are changing data science teamwork by allowing real-time code and model collaboration, version control, easy sharing of insights and visuals, and centralised data management. Tools like Databricks and Google Colab make this collaboration simple.
Measurable Computing Resources – Cloud environments let data scientists easily scale computing resources as needed, including adjusting power, using powerful GPUs, and leveraging cluster computing. This flexibility helps handle larger and more complex problems without traditional infrastructure limits.
Combining data science with cloud environments will keep boosting innovation and efficiency as we move forward. Edge computing and IoT data processing are changing how we work with data. Ethical AI and responsible data practices are becoming more important, and automated machine learning and advanced analytics are making things easier and more efficient. We can expect continued growth and change in the future of data science
Moreover, quantum computing and the ongoing shift to cloud-based data science will bring even more exciting opportunities shaping the future of data science . To stay ahead, data scientists and organisations need to stay updated and adaptable. Going with these new technologies, we can unlock new opportunities, bring innovation, and work on big challenges, creating a smarter, more data-driven world.
If you are interested in working in your field, consider taking data science courses taught by industry experts.